Blog
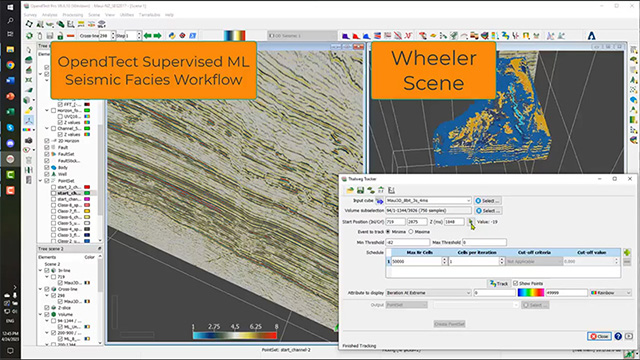
Machine Learning Workflows - Supervised AI Seismic Facies
- Written by: Paul de Groot
Previously, in our series on OpendTect Machine Learning workflows, we showed an unsupervised workflow for seismic facies analysis. That workflow clustered seismic waveforms to generate a segmentation volume consisting of 50 different segments enabling detailed interpretation of seismic facies.
Today, we show one of several possible workflows in OpendTect using supervised learning. This workflow uses the Thalweg tracker for labeling target positions. In total 8 different label sets were created representing positive and negative amplitude classes of meandering channels, unconfined channels, splays and floodplains.
Read more: Machine Learning Workflows - Supervised AI Seismic Facies
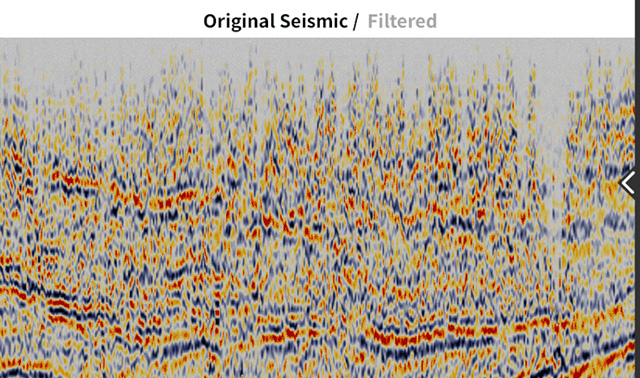
Free webinar on Approaches to Data Conditioning in OpendTect
- Written by: Marieke van Hout
Thursday, May 25th at 4 pm CET
Explore the diverse approaches to handling noisy or problematic seismic data in OpendTect. From trusted attributes to cutting-edge Machine Learning techniques.
Read more: Free webinar on Approaches to Data Conditioning in OpendTect
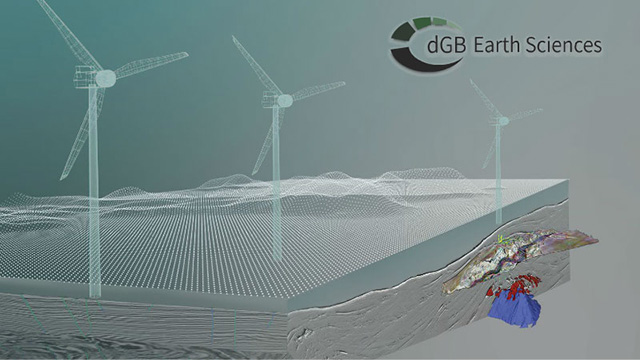
dGB provides expert support for Doordewind Wind Farm Zone
- Written by: Marieke van Hout
Exciting News! dGB Earth Sciences Partners with BLIX Consultancy & GEO2 Engineering B.V. to provide expert support for Doordewind Wind Farm Zone
Read more: dGB provides expert support for Doordewind Wind Farm Zone
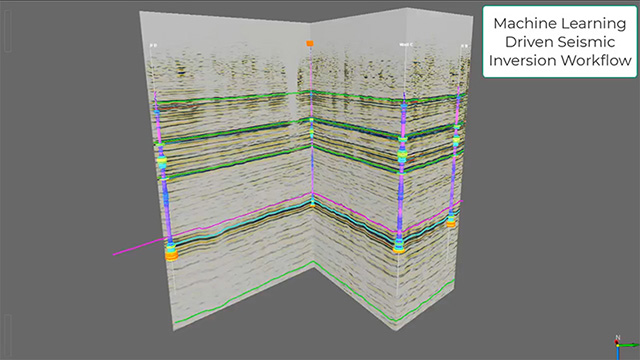
Machine Learning Workflows - Seismic Inversion using AI - Machine Driven Seismic Inversion Workflow
- Written by: Paul de Groot
Today, in our series on OpendTect Machine Learning workflows, we show a workflow for rock property prediction using real wells.
This workflow has many variations. You can train on real or synthetic seismic data to predict well log properties of interest.
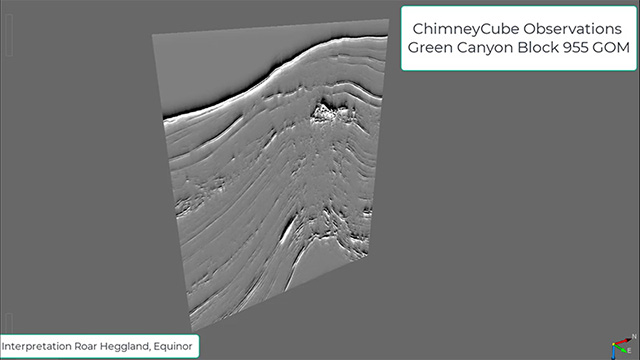
Machine Learning Workflows - De-risking charge and seal issues with AI - Neural Network Chimney Cube
- Written by: Paul de Groot
Today, in our series on Machine Learning Workflows, we go back to the origins of the OpendTect Machine Learning platform. OpendTect started life as a neural network-based seismic pattern recognition and attribute processing system. The primary goal of the original system was to create Chimney Cubes for fluid migration path interpretation. The software was used for geohazard interpretation and for de-risking hydrocarbon charge and seal problems.
The video shows a chimney interpretation study in the Gulf of Mexico by Roar Heggland of Equinor.
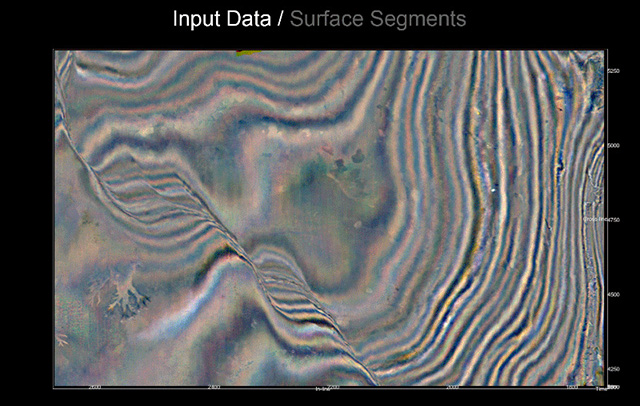
Surface Segments – The art of removing data to improve the signal to noise of your interpretation objective
- Written by: Marieke van Hout
Free webinar, Thursday 20 April 4 PM CET
Join us for an exclusive webinar as we unveil our latest innovative commercial plugin – the "Surface Segments" plugin
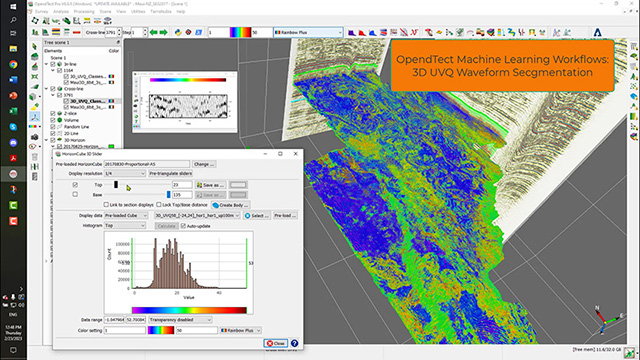
Machine Learning Workflows - Fast and Simple Seismic Facies Analysis - 3D UVQ Waveform Segmentation
- Written by: Paul de Groot
OpendTect Machine Learning supports supervised and unsupervised workflows for seismic facies analysis.
Today’s post discusses an unsupervised workflow. The 3D UVQ Waveform Segmentation workflow is the 3D variant of the Quick UVQ workflow that we discussed earlier in this series on OpendTect ML workflows. In Quick UVQ we segment (cluster) seismic waveforms (trace segments) around a mapped horizon into a user-defined number of segments. A typical number of segments in Quick UVQ is 10.
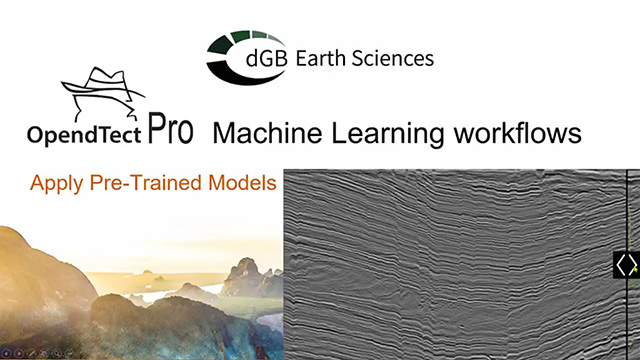
Machine Learning Workflows - Ready to go AI workflows - Apply Pre-trained Model
- Written by: Marieke van Hout
OpendTect’s Machine Learning platform is developed for three types of geo-scientists:
- Operational,
- Experimental and
- Research.
Read more: Machine Learning Workflows - Ready to go AI workflows - Apply Pre-trained Model
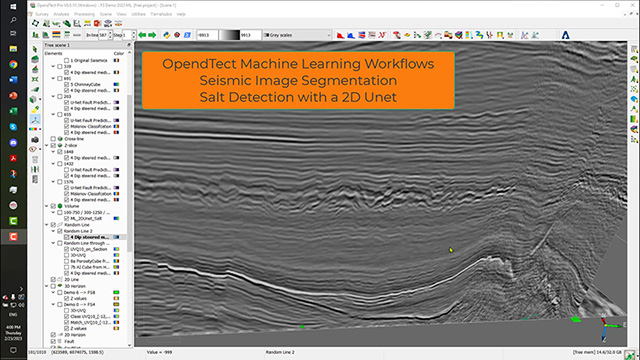
Machine Learning Workflows - Using AI for Salt Detection
- Written by: Paul de Groot
This is the second post in our series on OpendTect Machine Learning workflows. This time we show how to extract a salt body from 3D seismic. The ML workflow is called “Seismic Image Segmentation.” We train a 2D Unet (128x128 samples) to transform a seismic image into an image with values between 0 (no salt) and 1 (salt). The labels for training the Unet are created from interpretations of salt boundaries on a few sections. The trained Unet generates a new volume with values between 0 and 1 from which a 3D salt body is extracted.
Read more: Machine Learning Workflows - Using AI for Salt Detection