Blog
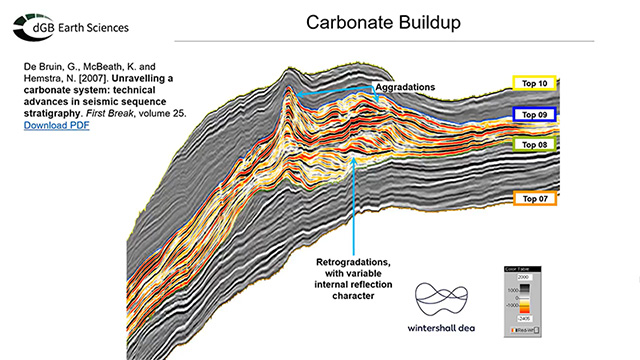
Carbonate Buildup Interpretation
- Written by: Paul de Groot
In seismic sequence stratigraphic studies, we analyze seismic reflection patterns in the context of a chronostratigraphic framework. In OpendTect, such frameworks are defined by a HorizonCube, which consists of a dense set of horizons representing Relative Geologic Time. The SSIS (Sequence Stratigraphic Interpretation System) plugin is an add-on that uses HorizonCube input to decompose depositional systems over geologic time.
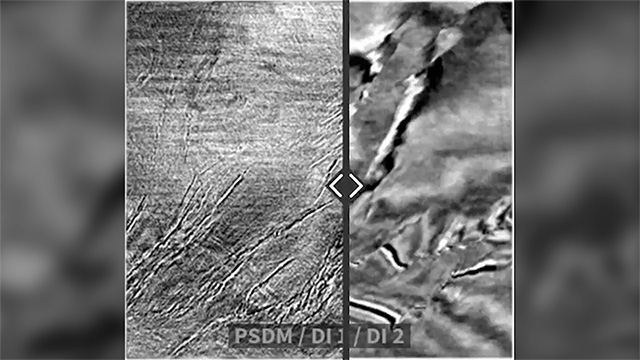
Seismic Interpretation Services
- Written by: Paul de Groot
Next to developing OpendTect we also offer advanced seismic interpretation services. Our experts have worked on data sets from around the globe. We have extensive experience in hydrocarbon exploration, field development, time-lapse monitoring, unconventional plays and geothermal studies.

OpendTect patch release 6.6.9
- Written by: Arnaud Huck
Dear OpendTect Users,
We have made a patch release for our latest stable version: OpendTect 6.6.9, which is now available for installation/update. We are proud to announce that this release is the first one fully certified by Red Hat.
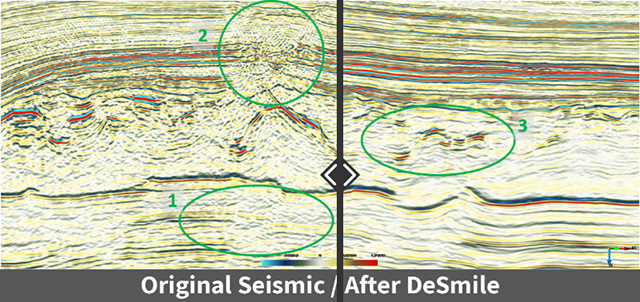
Improving interpretability with Machine Learning
- Written by: Paul de Groot
Today, we show another great example of how you can quickly improve the interpretability of seismic data by applying a trained Machine Learning model.
DeSmile is one the models from Lundin-GeoLab (nowadays AkerBP) that is available in OpendTect’s growing library of trained ML models
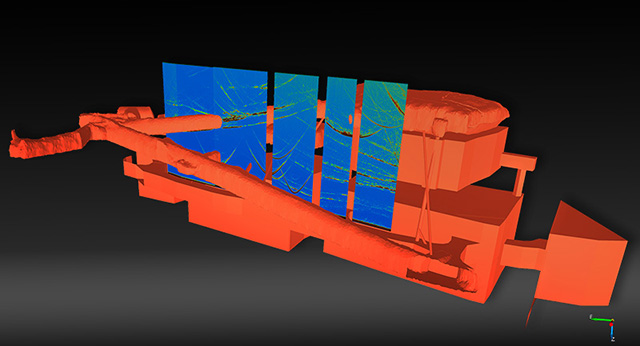
OpendTect is now STL compatible thanks to BGE sponsoring
- Written by: Marieke van Hout
Our latest OpendTect v6.6.8 supports STL format. This is a format used in the Computer Aided Design industry to design 3D objects. It is used in the Mining industry to show subsurface constructions such as mines. A nice feature (not shown) in OpendTect is that you can display attributes on the surface of the 3D body. For example displaying fault attributes would show where faults are intersecting the structure
Read more: OpendTect is now STL compatible thanks to BGE sponsoring
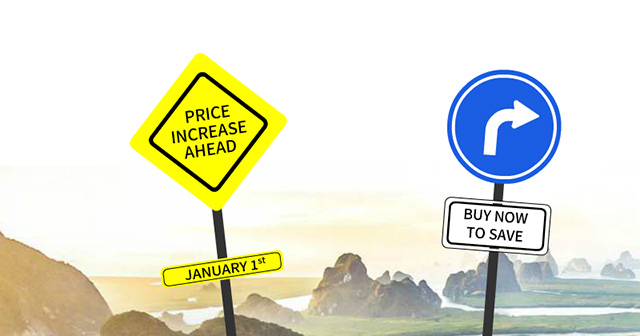
Upcoming Price Increase
- Written by: Paul de Groot
We’ve held our prices pretty much constant for a considerable time now but due to spiraling inflation we will be increasing our prices by 10% in the new year.
The updated prices will allow us to maintain and improve our standards for excellence. We will keep pushing the boundaries of technology, improving OpendTect's performance and support, so that we can continue to give you the very best value for money.
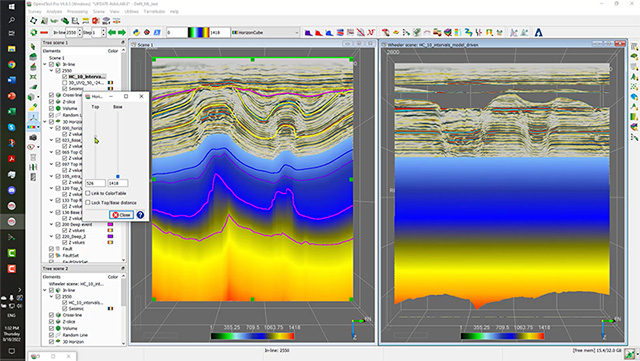
High-Resolution 3D Waveform Segmentation
- Written by: Paul de Groot
In April 2022, Friso Brouwer of I^3 GEO, conducted an OpendTect webinar in which he showed how high-resolution 3D waveform segmentation can be used for quick geomorphological analysis.
In this post, we show another application of Friso’s workflow. We use the Delft survey, which is freely available from TerraNubis, our cloud portal. Delft is one of several free projects that do not check for license keys, meaning you can run OpendTect Pro and commercial plugins on this dataset.
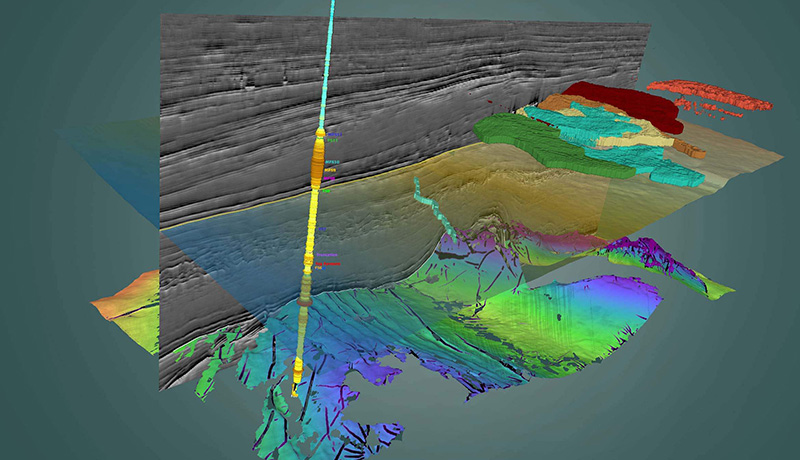
5 Online Training Courses in one week - 26th to 30th September
- Written by: Lloyd van Hout
In the week of 26th to 30th of September we will be organising a different online training course every day. The courses will be held from 9:00 AM to 4:00 PM CET. You can sign up for each course for only 100 USD per day. We have a special 50% off for academics. If you are an academic please email
Click on the subject to sign up for the Training Course(s) of your liking.
Read more: 5 Online Training Courses in one week - 26th to 30th September
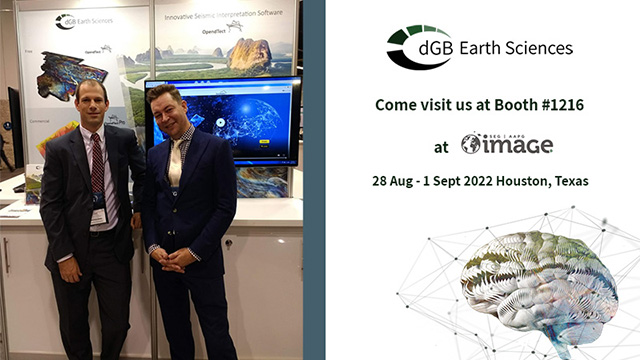
dGB Earth Sciences at IMAGE 2022 - Houston
- Written by: Marieke van Hout
Come see us at this year's International Meeting of Applied Geoscience and Energy August 28th - September 1st, 2022 in Houston Texas Booth #1216
Kristoffer Rimaila and Nanne Hemstra will present live demos on the latest developments in OpendTect. They will also showcase version 7, which will be released later this year.